Project Overview
Project Purpose
The project purpose is to develop a sophisticated Large Language Model (LLM) capable of summarizing Arabic legal texts using a specific template, enhancing accessibility and efficiency in legal document processing.
Dataset
We've compiled a comprehensive dataset of 25,000 legal cases from Morocco, originally in PDF format. To make this data usable, we employed Optical Character Recognition (OCR) for accurate text extraction.
Data Annotation and Gold Standard
Leveraging the advanced capabilities of GPT-4, we generated summaries of the extracted texts following a predefined template. These summaries serve as the gold standard for model training, balancing efficiency with high-quality annotations.
Model Fine-Tuning
LLaMA 3.2 3B Instruct LLM is choosen for fine-tuning, utilizing the gold standard summaries. LLaMA was selected for its strong performance, Arabic language comprehension, and open-source nature, making it ideal for the specialized task.
Results
Post fine-tuning evaluation on unseen test data revealed significant improvements:
- Performance boost ranging from 10% to 26%
- Impressive results considering the model size (3 billion parameters)
- Serves as a robust proof of concept, with potential for further enhancements using larger models
Knowledge Graph
To maximize the value of the summarized and extracted data, we constructed a knowledge graph. This graph visually represents the intricate relationships between cases, based on extracted properties such as case topics and applied legal principles. Explore this interactive tool in the "Created Knowledge Graph" tab.
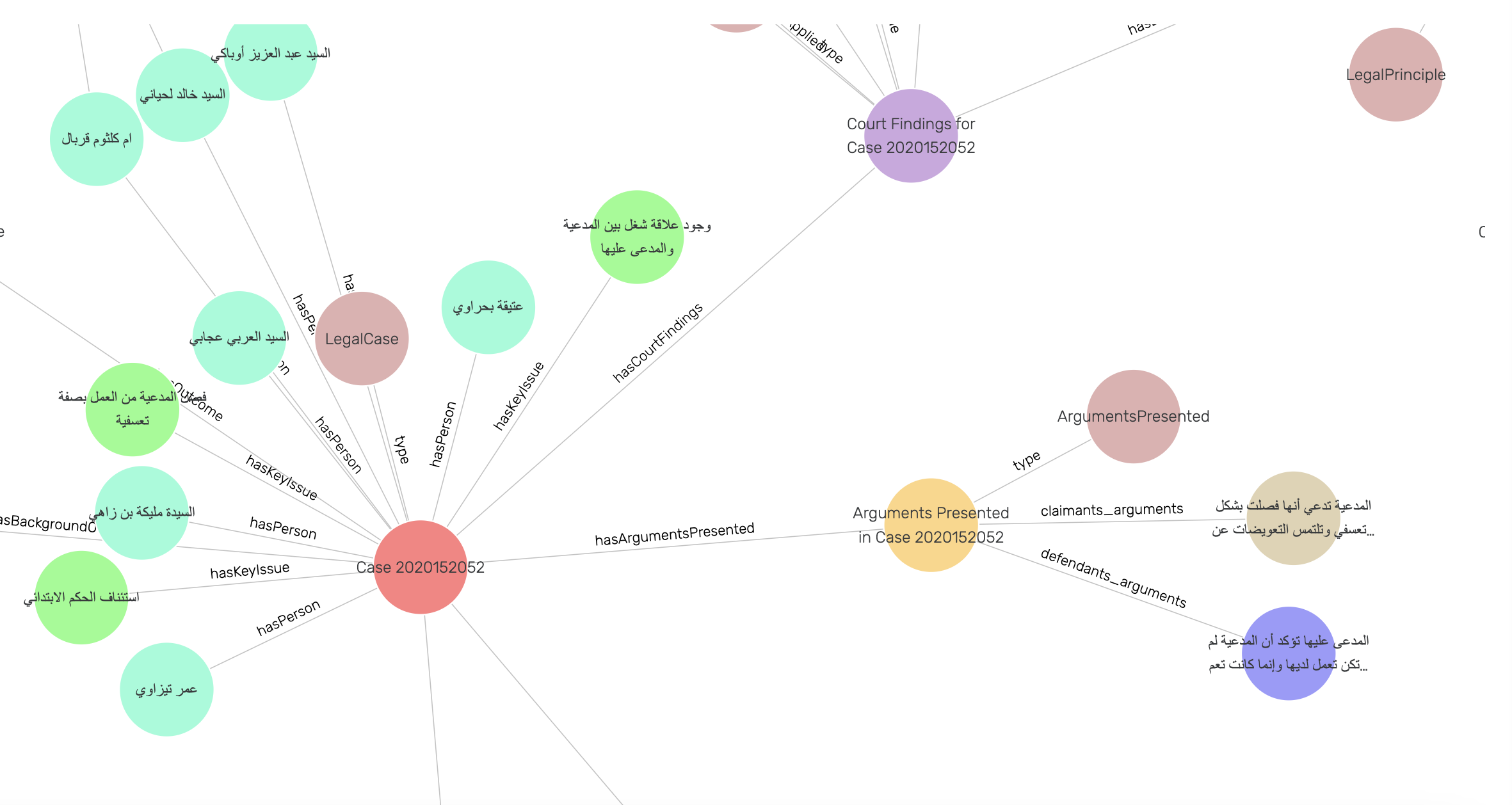
Model Availability
The fine-tuned model is now publicly accessible on Hugging Face. You can interact with and explore the model's capabilities through the "Chat with Fine-tuned Model" tab.
Data Extraction Process
Data Source
The primary data source for this project is the Judicial Portal of the Kingdom of Morocco, which provides public access to a vast repository of legal cases.
Web Crawling
A custom web crawler was developed and deployed to systematically download 25,000 PDF files of available legal cases from the portal. This automated process ensured efficient and comprehensive data collection.
Optical Character Recognition (OCR)
The collected PDF files were primarily scanned documents, necessitating an OCR approach for text extraction. The process involved:
- Converting each PDF page to an image format
- Applying OCR techniques to each image
- Compiling the extracted text from all pages into cohesive text files
Text Refinement
To address potential OCR errors and issues caused by document watermarks, an additional refinement step was implemented:
- The extracted text was processed through GPT-4
- GPT-4 was tasked with identifying and rewriting sentences that lacked coherence or contained grammatical errors
- This step significantly improved the overall quality and readability of the extracted text
Sample PDF Document
Below is a sample of an original PDF file from the dataset:
Extracted Information
The following JSON data represents the information extracted from the sample PDF, structured according to our specific template:
Related Resources
The scripts used in the data extraction process can be found here
Model Fine-Tuning Process
Model Selection: LLaMA 3.2 3B Instruct
The LLaMA 3.2 3B Instruct model was chosen for this project due to several compelling reasons:
- Strong performance in multilingual tasks, including Arabic
- Compact size (3 billion parameters) allowing for efficient fine-tuning
- Open-source nature, facilitating customization and deployment
- Instruction-following capabilities, crucial for the summarization task
- Utilization of the LLaMA 3.2 Instruct template for improved performance, leveraging the model's pre-training on instruction-following tasks
Prompt Template for Dataset Preparation
A carefully crafted prompt template was used to prepare the training dataset, ensuring consistency and quality in the fine-tuning process. The complete prompt structure is as follows:
This prompt structure aligns with the LLaMA 3.2 Instruct template, optimizing the model's ability to understand and generate appropriate responses for the legal summarization task.
Fine-Tuning Setup
The fine-tuning process leveraged several advanced technologies to optimize performance and efficiency:
- DeepSpeed: Employed for distributed training, enabling faster and more memory-efficient fine-tuning
- PEFT (Parameter-Efficient Fine-Tuning): Utilized LoRA (Low-Rank Adaptation) for efficient adaptation of the model
- Hugging Face Transformers: Used for model loading and tokenization
- TRL (Transformer Reinforcement Learning): Implemented for supervised fine-tuning
Hardware Optimization
The fine-tuning process was optimized based on available hardware:
- Utilization of bfloat16 precision on compatible GPUs for improved training speed and memory efficiency
- Implementation of Flash Attention 2 on supported hardware for faster attention computations
- Fallback to float16 precision and standard attention mechanisms on older GPU architectures
Training Configuration
The training process was carefully configured to balance performance and resource utilization:
- Batch size optimization using gradient accumulation (32 steps) to simulate larger batch sizes
- Learning rate set to 2e-4 with the AdamW optimizer for effective weight updates
- Implementation of gradient checkpointing to reduce memory usage during backpropagation
- Utilization of DeepSpeed Stage 2 optimization for efficient distributed training
LoRA Configuration
The LoRA setup was optimized for the legal summarization task:
- Rank (r) set to 64 for a good balance between model capacity and efficiency
- Alpha value of 16 to scale the LoRA updates appropriately
- Target modules included key, query, value, and feed-forward layers for comprehensive adaptation
Training Process
The training was executed with the following key aspects:
- 3 epochs of training to balance learning and overfitting prevention
- Regular evaluation (every 500 steps) to monitor performance
- Model checkpoints saved every 500 steps with a limit of 2 saved checkpoints
- Integration with Weights & Biases for comprehensive training monitoring and visualization
Post-Training
After the fine-tuning process:
- The best-performing model was automatically selected based on evaluation metrics
- The fine-tuned model was saved and made available for deployment and further testing
- The model was uploaded to Hugging Face for easy access and potential community contributions
Weights & Biases
Weights & Biases report for the fine tuning process:
Related Resources
The scripts used in the fine tuning process can be found here
Model Evaluation
Performance Overview
The fine-tuning process resulted in significant improvements across multiple evaluation metrics, with performance increases ranging from 10% to 26%. These results are particularly promising considering the model size of 3 billion parameters, demonstrating the effectiveness of the fine-tuning approach.
Evaluation Metrics
Multiple complementary metrics were used to comprehensively evaluate the model's performance:
BERTScore F1
This metric evaluates semantic similarity using contextual embeddings, making it particularly suitable for Arabic text evaluation. It shows significant improvements across all fields, with the highest gains in court information (0.9514 from 0.9056) and legal principles (0.8181 from 0.7133).
Relevance: BERTScore's contextual nature makes it ideal for assessing legal text where precise meaning is crucial.
Semantic Similarity
Measures the overall semantic closeness between generated and reference summaries. The fine-tuned model showed marked improvement, particularly in court details (0.9709 from 0.9399) and legal principles (0.9046 from 0.8350).
Relevance: Essential for ensuring the preserved meaning in legal summaries, where accuracy is paramount.
WMD (Word Mover's Distance) Similarity
Calculates the minimum distance required to transform one text into another, considering word embeddings. Notable improvements in court information (0.9265 from 0.8555) and legal principles (0.7496 from 0.6316).
Relevance: Measures the minimum "travel distance" between word embeddings. Captures semantic similarity well, even with different word choices. Given the nature of legal summarization, where the generated summary might use different phrasing to convey the same meaning, WMD is likely to be more forgiving and give higher similarity scores for semantically similar but differently worded phrases
BLEURT Score
A learned metric that correlates well with human judgments. Significant improvements across all fields, with court information reaching 0.9165 from 0.8281.
Relevance: Provides a human-like evaluation of summary quality, crucial for legal document summarization. Captures nuanced differences in meaning. Good at assessing fluency, coherence, and factual consistency.
JSON Validity
Measures the structural correctness of the generated JSON output. Both models maintained a high validity score.
Relevance: Critical for ensuring the generated summaries maintain the required structured format for downstream processing.
Detailed Performance Comparison
Base Model Performance
Key | BERTScore F1 | Semantic Similarity | WMD Similarity | BLEURT Score | Score |
---|---|---|---|---|---|
Court | 0.9056 | 0.9399 | 0.8555 | 0.8281 | - |
Main Case Topic | 0.6631 | 0.8037 | 0.5788 | 0.3977 | - |
Overview | 0.5726 | 0.7399 | 0.4930 | 0.2115 | - |
Relevant Dates | 0.8180 | 0.8999 | 0.5038 | 0.3621 | - |
Key Issues | 0.6205 | 0.7695 | 0.5112 | 0.2116 | - |
Claimant's Arguments | 0.5531 | 0.7467 | 0.4812 | 0.2232 | - |
Defendant's Arguments | 0.5307 | 0.7347 | 0.4734 | 0.1761 | - |
Evidence Reviewed | 0.5564 | 0.7254 | 0.4821 | 0.2259 | - |
Rulings Made | 0.5922 | 0.7551 | 0.5105 | 0.2839 | - |
Legal Principles Applied | 0.7133 | 0.8350 | 0.6316 | 0.4954 | - |
Final Decision | 0.6221 | 0.7654 | 0.5346 | 0.3464 | - |
Implications | 0.5311 | 0.7460 | 0.4736 | 0.1720 | - |
JSON Validity | - | - | - | - | 0.95 |
Fine-tuned Model Performance
Key | BERTScore F1 | Semantic Similarity | WMD Similarity | BLEURT Score | Score |
---|---|---|---|---|---|
Court | 0.9514 | 0.9709 | 0.9265 | 0.9165 | - |
Main Case Topic | 0.7616 | 0.8612 | 0.6733 | 0.5664 | - |
Overview | 0.6801 | 0.8348 | 0.5474 | 0.4414 | - |
Relevant Dates | 0.8597 | 0.9281 | 0.5930 | 0.4809 | - |
Key Issues | 0.6926 | 0.8318 | 0.5579 | 0.3342 | - |
Claimant's Arguments | 0.6328 | 0.8257 | 0.5171 | 0.4114 | - |
Defendant's Arguments | 0.6180 | 0.8237 | 0.5128 | 0.3843 | - |
Evidence Reviewed | 0.6400 | 0.8195 | 0.5128 | 0.4197 | - |
Rulings Made | 0.6852 | 0.8455 | 0.5572 | 0.4870 | - |
Legal Principles Applied | 0.8181 | 0.9046 | 0.7496 | 0.6648 | - |
Final Decision | 0.7148 | 0.8624 | 0.5889 | 0.5424 | - |
Implications | 0.6313 | 0.8425 | 0.5195 | 0.4336 | - |
JSON Validity | - | - | - | - | 0.99 |
Key Improvements:
- implications accuracy improved by 26.16%
- Court information accuracy improved by 4.58%
- Legal principles identification improved by 10.48%
- Case overview quality improved by 10.75%
- Arguments comprehension improved by approximately 9%
Evaluation Significance
The comprehensive evaluation approach using multiple metrics provides a robust assessment of the model's improvements:
- Semantic metrics ensure accuracy in legal content representation
- Structure validation confirms reliable data processing capabilities
- Consistency across metrics demonstrates genuine performance improvement
- Field-specific improvements align with legal domain requirements
Related Resources
The scripts used in the evaluation process can be found here
Knowledge Graph Creation
Ontology Development
Based on the structured information extracted from legal documents and stored in JSON format, a comprehensive ontology was developed. This ontology closely follows the properties defined in the JSON template, ensuring a seamless transition from extracted data to knowledge representation.
Knowledge Graph Construction
The knowledge graph leverages the rich information embedded in the extracted data, transforming it into a network of interconnected entities and relationships. This graph provides a visual and queryable representation of the intricate connections between legal cases, based on various extracted properties such as:
- Case topics
- Applied legal principles
- Involved parties
- Court decisions
- Relevant dates
Graph Database Implementation
The knowledge graph is implemented and maintained in a GraphDB instance. The user will be able to explore the graph visually and perform SPARQL queries.
SPARQL Query Capabilities
One of the key features of the knowledge graph is its ability to support SPARQL queries. This powerful querying capability enables users to extract specific information from the graph, such as:
- Retrieving all cases from a specific court
- Identifying cases that share common legal principles
- Tracing the evolution of legal interpretations over time
- Analyzing patterns in court decisions across different jurisdictions
These queries provide valuable insights and facilitate in-depth analysis of legal trends and precedents.
Knowledge Graph Demo
Below is a demonstration of the created knowledge graph, showcasing its structure, capabilities, and potential applications in legal research and analysis:
Related Resources
The scripts used in the knowledge graph creation process can be found here
Created Knowledge Graph
Interactive Knowledge Graph Visualization
Below is an interactive visualization of the created knowledge graph. This graph represents the complex relationships between legal cases, principles, and entities extracted from the dataset. You can explore the graph by zooming, panning, and clicking on nodes to see more details.
Fine-tuned Model Interaction
Chat with the Legal Assistant
Interact with the fine-tuned legal assistant model. This model is specialized in summarizing and analyzing legal texts in Arabic.